The Role of Data Analytics in Modern Product Management: Tools, Techniques, and Trends
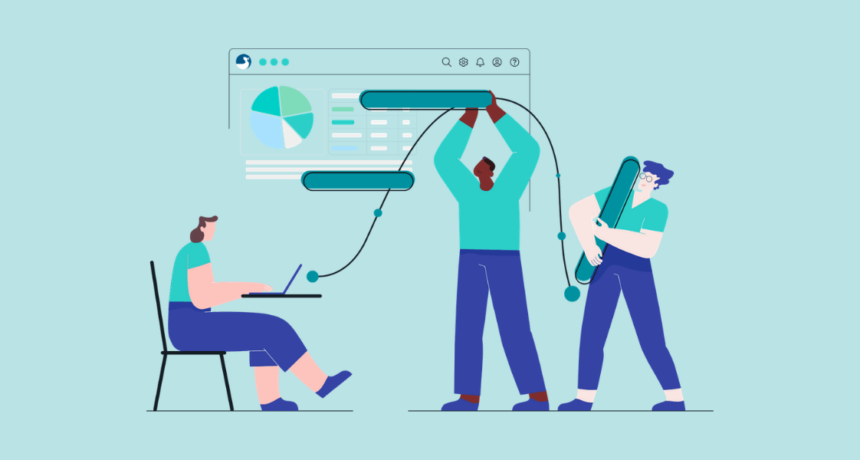
Contemporary product management has moved away from making decisions based on instinct towards a data-enabled solution where insights inform every strategic decision. The power to monitor user behavior, forecast market direction, and iterate on products in real-time has become a distinguishing element for business success. The proliferation of real-time data, along with improvements in AI and ML, has given companies the power to make decisions informed at a pace that has never been witnessed before.
With more than eight years of experience in running cross-functional teams, using analytics platforms, and designing scalable product architectures, I have spent much time working to integrate data analytics into product design. At Winaxis Private Limited, I drove enterprise-level data integration, automated decision-making dashboards, and created AI-driven models that were able to radically enhance customer interaction. At WebCodeGenie Technology, I specialized in the optimization of data-driven product roadmaps by collaborating with engineering and marketing teams in developing predictive models for pricing strategy and customer segmentation. This article investigates the contribution of data analytics to contemporary product management, outlining its influence through the product lifecycle, tools and techniques that inform decision-making, upcoming trends, and the issues that organizations need to overcome to remain competitive.
Understanding Data Analytics in Product Management
Data analytics in product management refers to the structured approach of collecting, processing, and interpreting data to optimize decision-making. In the past, product managers made use of market intuition, user anecdotes, and shallow competitive analysis. Nowadays, insights driven by data enable product teams to accurately measure how users interact with the product, measure product performance, and implement changes correctly.
Having spearheaded various projects aimed at real-time analytics and predictive modeling, I understand how organized insights decrease risk and enhance product-market fit. Organizations that incorporate real-time data analysis into their workflows gain a competitive advantage through faster iteration, customer need anticipation, and adaptive reaction to market fluctuations. Using Power BI and SQL-based reporting, I have enabled teams to switch from reactive analysis to predictive, future-oriented strategies.
Data Analytics Across Product Lifecycle Stages
Research & Discovery: Understanding customer pain points is critical to creating products that address actual problems in the real world. At Winaxis, I spearheaded data analysis initiatives that employed SQL-driven reporting to divide users by behaviour patterns. These learnings informed product improvements so that new features solved genuine user needs and not speculation.
Design & Development: Product development should be incremental and subject to ongoing testing and verification using A/B testing, heatmaps, and user behavior analysis. At WebCodeGenie Technology, I collaborated with UX designers and engineers to implement analytics solutions such as Hotjar and FullStory. These solutions offered session replays and behavior heatmaps, allowing our team to optimize product usability. We decreased bounce rates by more than 20% and increased customer engagement through embedding these insights.
Launch & Iteration: A successful product launch is gauged in terms of adoption rates, user activation, and retention. At Winaxis, I set up automated reporting channels to monitor post-launch behavior. This gave leadership real-time insights into customer interactions, feature adoption levels, and revenue effect, enabling fast decision-making and iteration.
Scaling: Predictive analytics is also key to demand forecasting, infrastructure optimization, and efficient resource allocation. Through experience on credit bureau integration projects for Highmark and Equifax, I have had the opportunity to apply predictive models to improve financial risk analysis. The models enhanced the accuracy of decision-making, enabling financial institutions to improve credit underwriting and fraud detection processes.Â
Key Tools in Data Analytics for Product Managers
Business analytics software like SQL, Power BI, and Tableau enable organizations to aggregate voluminous data sets, detect trends, and make automated choices. At Winaxis, I created KPI dashboards that gave executive teams real-time performance data, cutting down manual reporting workloads by 50% and enhancing response times to business-critical changes.
User Engagement platforms like Google Analytics, Mixpanel, and Amplitude give detailed insights into customer interactions. I implemented Mixpanel at WebCodeGenie Technology to study retention patterns and feature uptake, and we saw a 15% boost in user engagement in six months.
Real-time analytics tools like Segment and Apache Kafka have revolutionized the way companies handle live event data. With these tools, product managers can monitor user activity in real time, offering instantaneous feedback for tweaks. On e-commerce sites, I used real-time event streaming to optimize recommendation engines, lowering cart abandonment rates and boosting conversion rates by more than 10%.
How Leading Companies Use Data Analytics
Netflix has established a worldwide benchmark for AI-based content suggestions. The service handles more than 100 billion data points every day, studying viewing behavior, user engagement, and content interest. Through collaborative filtering and deep learning algorithms, Netflix has a 75% engagement rate for recommended content, which is much lower than churn. Netflix runs A/B tests on thumbnail images, metadata, and viewing habits constantly to improve engagement.
Spotify utilizes AI-powered behavioural clustering to offer hyper-personalized playlists to its 456 million users. Through monitoring listening behavior, skip rates, and engagement lengths, the platform tunes its Discover Weekly and Release Radar playlists, boosting overall listening time per user by more than 30%. Spotify also employs real-time sentiment analysis to forecast user engagement, making recommendations more relevant.
Amazon depends on machine learning algorithms to drive its recommendation engine, impacting almost 35% of all purchases. Based on browsing history, purchase patterns, and session data, Amazon dynamically adjusts product placement, thereby boosting conversion rates. Inventory forecasting using AI assists the company in optimizing supply chain management and decreasing warehouse expenses and efficient product delivery.
Airbnb utilizes predictive analytics to refine pricing models, predict demand, and improve host-guest experiences. Through the study of seasonal trends, competitor listings, and booking habits, Airbnb dynamically modifies listing prices to drive the highest occupancy rates. The data-driven pricing has been shown to boost host earnings and enhance customer satisfaction.
Real-Time Data and AI Integration
The advent of real-time analytics and AI has redefined the way companies are taking data-driven decisions. AI-enabled models coupled with real-time data streams enable companies to dynamically automate personalization, churn prediction, and product performance optimization. Apache Kafka and Segment make event-driven architectures seamless in nature, where product teams are able to respond in real-time to changes in customer behaviour.
AI/ML platforms like OpenAI, AWS SageMaker, and Google Vertex AI enable the deployment of predictive models that optimize segmentation strategies, recommenders, and decision-making. By incorporating ML-based sentiment analysis in customer feedback loops, companies can actively catch potential issues, enhance response times, and boost loyalty among customers.
Conclusion
Data analysis is now a key corner stone of contemporary product management, allowing businesses to make data-driven decisions that increase customer satisfaction, optimize product performance, and facilitate business success. As AI analytics continues to advance, product managers need to adopt automation, predictive insights, and instant decision-making in order to remain competitive.
From my background in fintech, e-commerce, and custom application development , I have witnessed firsthand the impact data analytics at scale has on building strong, top-performing products. As companies advance, those that incorporate data-driven methods into every phase of product development will shape the future of digital innovation. Those organizations that commit to sophisticated analytics now will spearhead tomorrow’s marketplace, establishing new industry standards for efficiency, flexibility, and customer success.
Source: The Role of Data Analytics in Modern Product Management: Tools, Techniques, and Trends